Assisted decision-making in medicine refers to using technology and other support systems to aid healthcare professionals in making clinical decisions. This approach involves collaboration between human expertise and computational tools to enhance medical decision-making accuracy, efficiency, and effectiveness.
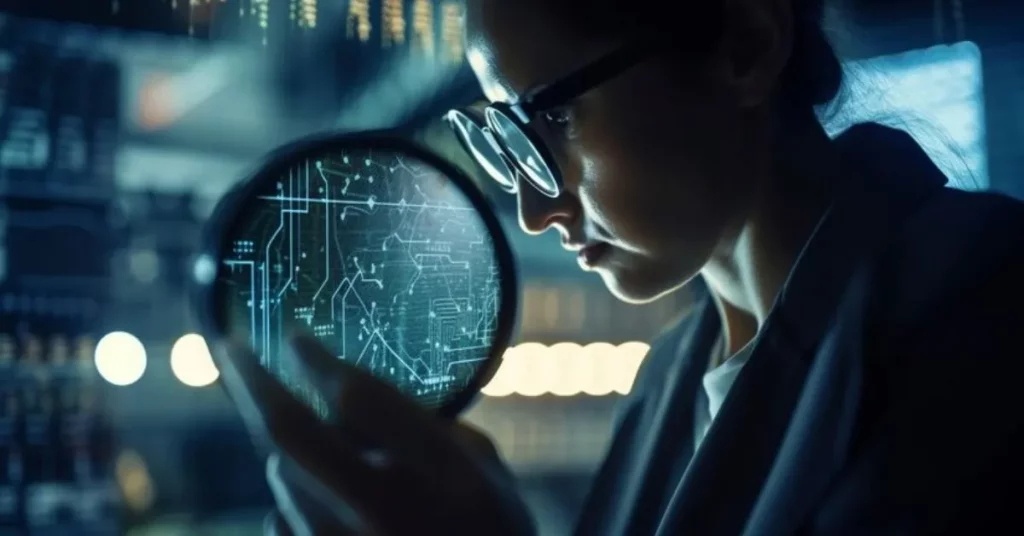
OpenSourceResearch collaboration (OSRC) identified some key aspects of assisted decision-making in medicine these are:
- Decision Support Systems (DSS): These computer-based systems provide healthcare professionals with information and knowledge to assist decision-making. DSS can analyze patient data, medical literature, and other relevant information to offer recommendations or predictions.
- Artificial Intelligence (AI) and Machine Learning (ML): AI and ML algorithms can analyze large datasets to identify patterns, trends, and correlations that may not be immediately apparent to human practitioners. These technologies can predict patient outcomes, assist in diagnosis, and recommend treatment options.
- Clinical Decision Support (CDS): CDS systems provide healthcare professionals with relevant clinical knowledge and patient-specific information at the point of care. They can offer alerts, reminders, and suggestions based on best practices and evidence-based guidelines.
- Data Analytics: Analyzing healthcare data, including electronic health records (EHRs), can contribute to decision-making by providing insights into patient populations, disease trends, and treatment outcomes. This data-driven approach helps healthcare professionals make informed decisions.
- Telemedicine: Remote consultation and telehealth services can provide access to expert opinions and medical advice, especially in areas with limited healthcare resources. This can support healthcare professionals in making decisions by leveraging expertise from different locations.
- Genomic Decision Support: In fields such as oncology, genomic data is increasingly used to personalize treatment plans. Decision support systems can interpret genomic information to help guide treatment decisions based on the genetic profile of a patient’s tumor.
- Ethical Considerations: Assisted decision-making in medicine also involves considering ethical implications. Human oversight is crucial to ensure that decisions align with ethical standards and that technology respects patient autonomy and privacy.
While assisted decision-making can significantly enhance healthcare practices, balancing technology and human judgment is essential. Healthcare professionals remain responsible for interpreting the information provided by these systems and making the final decisions in the patient’s best interest. This vital message was presented several times during the workshop and course organized by OpenSourceResearch Collaboration (OSRC)
OpenSourceResearch collaboration and future research in this field
Assisted decision-making in medicine was rapidly evolving, with ongoing research in several key areas. Keep in mind that the information might have progressed since then. OpenSourceResearch Collaboration (OSRC) believes that the most important directions in which research in this field is heading are:
- Explainable AI (XAI): Enhancing the interpretability of AI and machine learning models is a crucial area of research. Researchers are working on developing models that make accurate predictions and explain their decisions, especially in complex medical scenarios. This is essential for gaining the trust of healthcare professionals and ensuring transparency in decision-making processes.
- Integration with Electronic Health Records (EHRs): Efforts are being made to seamlessly decision-support systems with electronic health records. This includes developing interoperable systems to access and analyze patient data in real-time to provide relevant and timely recommendations.
- Personalized Medicine and Genomic Decision Support: With the increasing availability of genomic data, research is focused on developing decision support tools that can interpret genetic information to guide personalized treatment plans. This involves understanding the unique genetic makeup of individuals and tailoring interventions accordingly.
- Real-Time Data Analytics: Researchers are exploring leveraging real-time data analytics for dynamic decision support. This includes using continuous monitoring devices, wearables, and other real-time data sources to provide healthcare professionals with up-to-date information for decision-making.
- Human-AI Collaboration: The emphasis on the collaboration between healthcare professionals and AI systems is growing. Research is focused on understanding how best to integrate AI tools into existing clinical workflows, ensuring that the decision-making process is a synergistic interaction between human expertise and machine intelligence.
- Ethical and Legal Frameworks: As AI plays an increasingly prominent role in healthcare decision-making, researchers are exploring these technologies’ ethical and legal implications. This includes issues related to patient privacy, consent, algorithm bias, and the overall ethical use of AI in healthcare.
- Cross-Disciplinary Collaboration: The field involves collaboration between computer scientists, healthcare professionals, ethicists, and other stakeholders. Research efforts are focused on fostering interdisciplinary collaborations to ensure that technological developments align with the needs and values of the healthcare community.
- Patient-Centered Approaches: There is a growing emphasis on involving patients in decision-making processes. Research explores ways to incorporate patient preferences, values, and input into decision support systems to ensure that healthcare decisions align with individual patient needs.
How can we accelerate research in this field?
Accelerating research in assisted decision-making in medicine involves fostering collaboration, promoting innovation, and addressing various challenges. Here are some strategies that OpenSourceResearch Collaboration (OSRC) suggests to accelerate research in this field:
Interdisciplinary Collaboration:
- Encourage collaboration between computer scientists, data scientists, healthcare professionals, ethicists, and other relevant experts.
- Establish interdisciplinary research teams to address complex challenges that require expertise from multiple domains.
Data Sharing and Standardization:
- Promote the sharing of healthcare data while respecting privacy and ethical considerations.
- Work towards standardizing data formats and protocols to facilitate interoperability between different systems and institutions.
Open Access and Open Source:
- Encourage open access to research findings and datasets to facilitate knowledge sharing.
- Promote developing and using open-source software and tools, allowing researchers to build upon each other’s work.
Public-Private Partnerships:
- Foster collaborations between academic institutions, healthcare organizations, and private companies to leverage resources and expertise.
- Public-private partnerships can accelerate the translation of research findings into practical applications.
Research Funding:
- Increase funding for research in assisted decision-making in medicine from government agencies, private foundations, and industry.
- Prioritize funding for projects that have the potential for significant impact on clinical practice and patient outcomes.
Technology Transfer and Implementation Science:
- Support initiatives that focus on effectively transferring technology from research labs to real-world clinical settings.
- Invest in implementation science research to understand how decision support systems can be integrated into routine healthcare practices.
Education and Training:
- Develop educational programs that train the next generation of researchers in the technical and clinical aspects of assisted decision-making.
- Promote cross-disciplinary training to foster a shared understanding between computer scientists and healthcare professionals.
Regulatory Frameworks:
- Work with regulatory bodies to establish clear guidelines for developing and deploying decision support systems in healthcare.
- Ensure that regulatory frameworks are adaptive to technological advancements while prioritizing patient safety and ethical considerations.
User-Centered Design:
- Prioritize user-centered design approaches to create intuitive decision support systems that align with healthcare professionals’ workflows.
- Involve end-users in the development process to gather feedback and improve the usability of tools.
Global Collaboration:
- Foster international collaboration to address global health challenges and share insights across healthcare systems.
- Collaborate on large-scale, multi-center studies to gather diverse datasets and improve the generalizability of research findings.
Ethical Considerations:
- Address ethical concerns related to data privacy, algorithm bias, and the responsible use of AI in healthcare.
- Engage in ongoing discussions with ethicists, policymakers, and the public to ensure that research aligns with ethical standards.
By implementing these strategies, the research community can work towards advancing the field of assisted decision-making in medicine more rapidly and effectively. It’s crucial to create an environment that encourages innovation, collaboration, and the translation of research findings into practical solutions that benefit patients and healthcare providers.